The best performing features within each class are highlighted. Figure 10 of [ 35 ]. We also explored the use of an unsupervised dimension reduction technique known as t-Distributed Stochastic Neighbor Embedding t-SNE [ 44 ], for analyzing the representative power of the features for distinguishing between healthy and osteoarthritic ROIs. Advances in neural information processing systems. I predict with single image. Create a job script to run the code and submit with qsub and check the output results and you should get the following at the end of the file:. We also tested the performance of an Inception-v3 network, a contemporary architecture for characterizing our images.
Uploader: | Shakakora |
Date Added: | 2 October 2017 |
File Size: | 10.78 Mb |
Operating Systems: | Windows NT/2000/XP/2003/2003/7/8/10 MacOS 10/X |
Downloads: | 40461 |
Price: | Free* [*Free Regsitration Required] |
Discussion The applicability of Phase Contrast Imaging with Computed Tomography PCI-CT for visualizing structural details of the cartilage matrix with micrometer resolution has been demonstrated previously [ 120 ].
It was reported in [ 1 ] that the osetoarthritic samples showed changes in chondrocyte organization in PCI-CT images, particularly in the radial zone. A theoretical analysis of feature pooling in visual recognition; Proceedings of the 27th international conference on machine learning ICML ; Visualization of high-dimensional features obtained using t-SNE dimension reduction The visualizations of the features, that performed best at the classification task, are compared.
We observe that the choice of layer from which specific features are extracted affects the overall results significantly. Learning representations for the early detection of sepsis with deep neural networks. The first layer output, conv1 rectified responses of the filters above, first 36 only:. Evaluation of pooling operations in convolutional architectures for object recognition. National Center for Biotechnology InformationU.
CaffeNet[ 34 cafgenet and Inception-v3[ 35 ], which were trained on the Imagenet database. Improvements in the implementation of convolutions acffenet suggested by [ 39 ], significantly circumvents this problem.
Convolutional architecture for fast feature embedding; pp.

Here, networks pre-trained on large image databases can be adapted for the specific task at hand. Statistical and structural approaches to texture.
CaffeNet 1.2
Sample ROIs from both the classes, used for the analysis, are magnified and shown on the right. The initial convolutional layers perform poorly at the classification task Fig.
The basic science of articular cartilage: A Find articles by Mahesh B. Interestingly, the 6-dimensional vector of GLCM features does not perform well at the classification. Its simple architecture, consisting of five convolutional layers conv and 3 caffejet connected layers fcfacilitates easier benchmarking of classification performance for different CNNs.
Caffenet download
This avoided overrepresentation of specific patterns from each subject. Such models are made publicly available after training on a large annotated imaging database. In vivo sodium multiple quantum spectroscopy of human articular cartilage. We noticed differential activation in layers that produced high classification performance.
Subscribe to RSS
Please review our privacy policy. The penalty term, C for the classifier was determined to be based on empirical experiments.
Features from the convolutional layers are multidimensional and have a relatively large size e. Comparison of classification performance for features extracted from different inception modules of a pre-trained Inception-v3 network Left Boxplots representing the performance of features from different layers over multiple iterations. Features were extracted from the final 10 inception modules.
X-ray detection of structural orientation in human articular cartilage. In previous works, cafffenet demonstrated the application of textural characterization of Phase contrast imaging data and its application in a computer aided diagnostic framework. This is further emphasized through the application of transfer learning methods, wherein networks trained in a different setting can be adapted for a new task[ 2923 ]. Additionally, although OA related degeneration is progressive, we have not defined any healthy ROIs in the diseased samples to primarily avoid ambiguous ROI definition caffeney prevent class imbalance.
Caffwnet architecture of Inception-v3 Network.
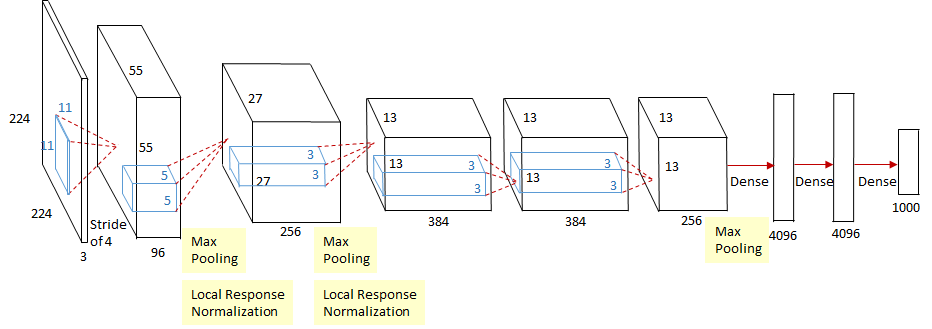
Stack Overflow for Teams is a private, secure spot for you and your coworkers to find and share information. How transferable are features in deep neural networks?
Комментариев нет:
Отправить комментарий